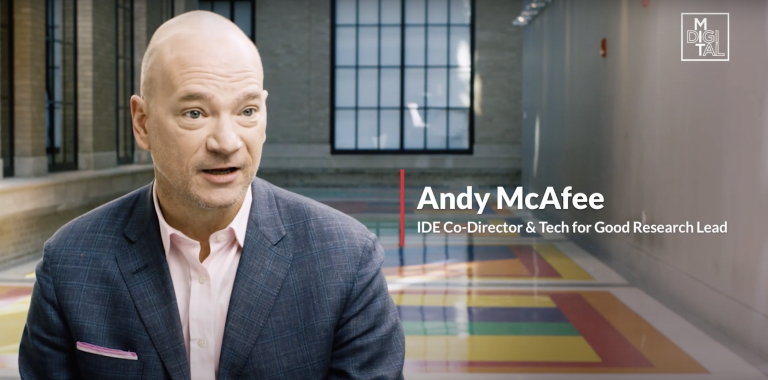
Latest Insights
More InsightsAI Automation and Vision Tasks
MIT IDE research lead, Neil Thompson, co-authored a paper estimating human worker displacement when AI is introduced for computer vision tasks. The study found the impact to be less dramatic than others have reported. Here are three takeaways:
How many?
23%
Percent of worker wages that would be cost-effective to automate with AI computer vision.
How much?
20%
Estimated decrease In annual computer vision AI costs.
When?
2044
Even with a 20% annual decrease in costs, it would take decades for computer vision tasks to become economically efficient for firms.
Research Groups
Featured Publications
Explore All PublicationsIDE on Medium
Read our blog publication for more about the impact of digital technology on business, the economy, and society.
Visit our Medium PublicationGenAI is Learning on the Job
Posted on: April 22, 2024
Good Data = Good GenAI
Posted on: April 15, 2024
Why Businesses are So Focused on Open Source AI
Posted on: April 09, 2024
What Does Ethical AI Look Like?
Posted on: April 02, 2024